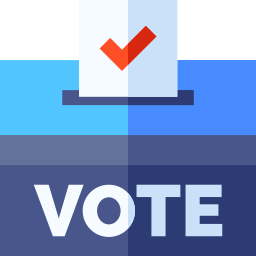
How can we increase participation of women in computing? How can we recruit and retain more women to study computing? Curricula are an obvious place to start. Understanding student motivations for their learning choices can help educators develop more effective programs of study. Join us to discuss a paper modeling women’s elective choices in computing by Steven Bradley, Miranda C. Parker, Rukiye Altin, Lecia Barker, Sara Hooshangi, Thom Kunkeler, Ruth G. Lennon, Fiona McNeill, Julià Minguillón, Jack Parkinson, Svetlana Peltsverger and Naaz Sibia from the Proceedings of the 2023 Working Group Reports on Innovation and Technology in Computer Science Education (ITiCSE). From the abstract:
Evidence-based strategies suggest ways to reduce the gender gap in computing. For example, elective classes are valuable in enabling students to choose in which directions to expand their computing knowledge in areas aligned with their interests. The availability of electives of interest may also make computing programs of study more meaningful to women. However, research on which elective computing topics are more appealing to women is often class or institution specific. In this study, we investigate differences in enrollment within undergraduate-level elective classes in computing to study differences between women and men. The study combined data from nine institutions from both Western Europe and North America and included 272 different classes with 49,710 student enrollments. These classes were encoded using ACM curriculum guidelines and combined with the enrollment data to build a hierarchical statistical model of factors affecting student choice. Our model shows which elective topics are less popular with all students (including fundamentals of programming languages and parallel and distributed computing), and which elective topics are more popular with women students (including mathematical and statistical foundations, human computer interaction and society, ethics, and professionalism). Understanding which classes appeal to different students can help departments gain insight of student choices and develop programs accordingly. Additionally, these choices can also help departments explore whether some students are less likely to choose certain classes than others, indicating potential barriers to participation in computing.
We’ll be joined by some of the co-authors of the paper who will give us a five minute lightning talk summary to kick-off our discussion. As usual we’ll be meeting on zoom, all welcome, joining details at sigcse.cs.manchester.ac.uk/join-us
References
- Steven Bradley, Miranda C. Parker, Rukiye Altin, Lecia Barker, Sara Hooshangi, Thom Kunkeler, Ruth G. Lennon, Fiona McNeill, Julià Minguillón, Jack Parkinson, Svetlana Peltsverger, Naaz Sibia (2023) ITiCSE-WGR ’23: Proceedings of the 2023 Working Group Reports on Innovation and Technology in Computer Science Education, Pages 196–226, DOI:10.1145/3623762.3633497
CC licensed image via flaticon.com