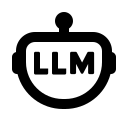
Large Language Models (LLMs) can help explain programming error messages and these explanations tend to improve as the models they are based on include more source code. However, it is unknown to what extent novice programmers are able to effectively utilise these automatically generated explanations to debug their programs, with tools like GitHub CoPilot and ChatGPT. Join us to discuss a paper on this by Eddie Antonio Santos and Brett Becker. This paper won a best paper award at UKICER.com earlier this year. We’ll be joined by the papers lead author, Eddie Antonio Santos, who’ll give a lightning talk to kick off our discussion. From the abstract:
The sudden emergence of large language models (LLMs) such as ChatGPT has had a disruptive impact throughout the computing education community. LLMs have been shown to excel at producing correct code to CS1 and CS2 problems, and can even act as friendly assistants to students learning how to code. Recent work shows that LLMs demonstrate unequivocally superior results in being able to explain and resolve compiler error messages—for decades, one of the most frustrating parts of learning how to code. However, LLM-generated error message explanations have only been assessed by expert programmers in artificial conditions. This work sought to understand how novice programmers resolve programming error messages (PEMs) in a more realistic scenario. We ran a within-subjects study with 𝑛 = 106 participants in which students were tasked to fix six buggy C programs. For each program, participants were randomly assigned to fix the problem using either a stock compiler error message, an expert-handwritten error message, or an error message explanation generated by GPT-4. Despite promising evidence on synthetic benchmarks, we found that GPT-4 generated error messages outperformed conventional compiler error messages in only 1 of the 6 tasks, measured by students’ time-to-fix each problem. Handwritten explanations still outperform LLM and conventional error messages, both on objective and subjective measures.
As usual, we’ll be meeting on zoom, all welcome, details at sigcse.cs.manchester.ac.uk/join-us.
References
- Eddie Antonio Santos and Brett A. Becker (2024) Not the Silver Bullet: LLM-enhanced Programming Error Messages are Ineffective in Practice, UKICER ’24: Proceedings of the 2024 Conference on United Kingdom & Ireland Computing Education Research DOI:10.1145/3689535.3689554